Cooking Data
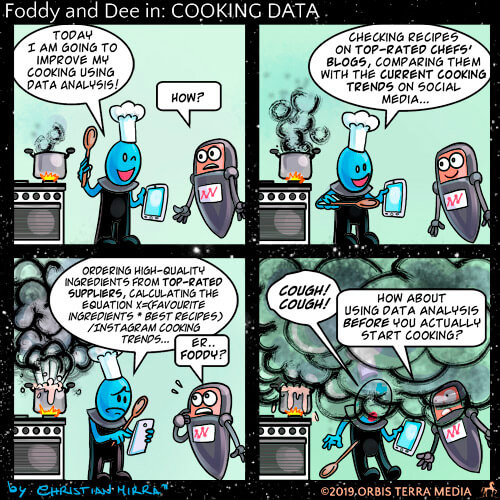
A lot has been said about the potential of big data in the marketing realm. We also know that, thanks to increasing digitalisation, many businesses find themselves with a wealth of data without necessarily knowing what to do with it or where its value lies.
The vagaries of big data’s potential often give rise to a slightly misleading idea that we can take an exploratory approach to discovering what our data might be able to tell us. While we might wish to leverage unstructured datasets, our approach must be anything but unstructured.
The very first step of any data analysis project must be to define our goals. Experimenting with data isn’t about not knowing what you want to do with it. You need to have a clear vision of the question you need answering first, and then work to understand which data can help you find that answer.
Why is my marketing campaign not going well? Do I already have the data I need to answer that question? How can I collate the data I need but don’t yet have? How long will it take? How am I going to chop, dice and serve up that data to devise new marketing strategies?
There is a reason data science is a science; you need to take a methodological approach to it.
There is a reason data science is a science; you need to take a methodological approach to it.
That’s not to say there isn’t an element of spontaneity and surprise in data science. The data you collate in the process of finding the answers you need can often take you in surprising directions. It can shed light on answers – and even questions – you might not have thought about previously.
You need to leave space within your scientific approach to allow these insights and to give yourself space to act on intuition. In this way, the experimentation comes later – when you adjust each iteration of the particular process you are aiming to improve. Used in this way, data helps you to gradually improve over time.
It’s good to try things out. And it’s ok to fail, especially if you can learn from it. However, even failure has to happen within certain parameters.
The problem with making things up as you go along without a clear goal in mind is that learning from what happens next isn’t easy, predictable or – often – useful.
However, if you set clear parameters first, even failure can be useful. And your work will help you uncover the real value of the big data you hold.